June 10, 2021
5 Major NLP Techniques To Power Up Your Communication Skills
Y Meadows
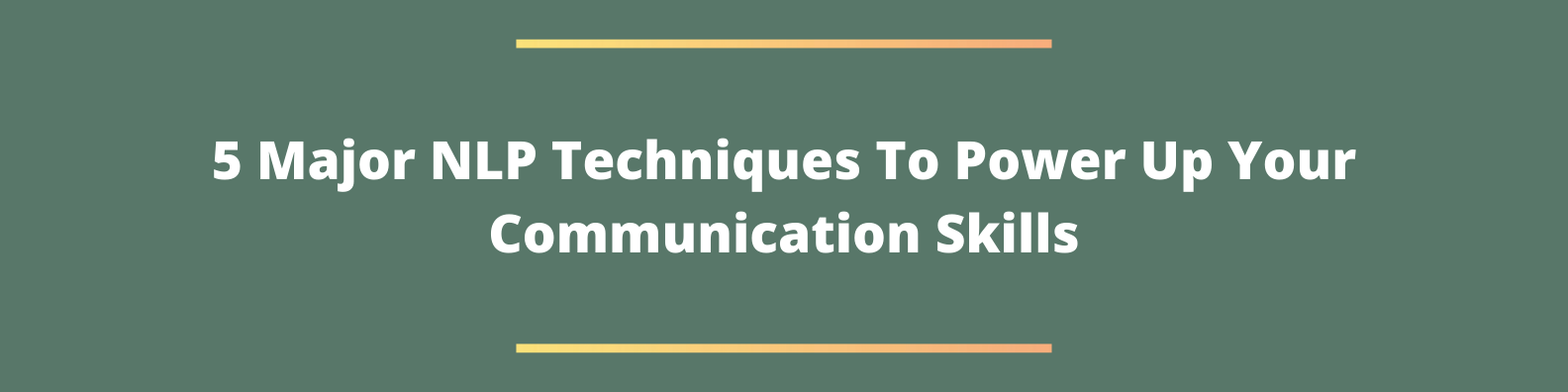
The domain of artificial intelligence has always imagined computers being able to emulate the abilities and functioning of the human mind. And human languages are considered one of the most significant achievements of humans throughout history that have tremendously expedited the progress of humanity. So, it comes as no surprise that there’s a lot of work being done to integrate language into the field of artificial intelligence, dominantly in the form of natural language processing, or NLP.
Nevertheless, computers are generally not designed to understand humans when they communicate as humans naturally do. Computers speak in code, using long lines of zeros and ones. On the contrary, humans are much more complicated, speaking in thousands of different languages and using sarcasm or phraseology. It seems like computers and humans can’t truly connect through language.

However, thanks to artificial intelligence and specific NLP techniques, nowadays, computers respond to our search terms, voice commands, complaints, and other types of human interaction with incredible accuracy to efficiently power up the communication skills and customer service efforts of companies that are ready to take the next step in terms of embracing innovative technology.
In this blog post, first, we’ll take a quick look at what natural processing language is and why it’s so important. Later, we’re going to focus on the five primary NLP techniques that can power up your company’s communication skills to provide outstanding customer experiences.
Defining Natural Language Processing
Often referred to as “text analytics,” natural language processing (NLP) is an intersection of artificial intelligence, computer science, and linguistics. The end goal of this groundbreaking technology is to help computers understand the content, nuances, and sentiment of what people write or say conversationally.
Thanks to NLP, computers nowadays can perfectly extract the information and insights contained in human language and organize them into their respective categories. For instance, whenever a user searches something like “most expensive cars in the world” on Google or another search engine, the search engine’s algorithm shows the most relevant articles, blogs, documents, and videos by utilizing specific NLP techniques.
If you’re eager to know more about NLP, the way it works, its most common applications, and real-world examples—feel free to read through our dedicated NLP blog post before we get down to the NLP techniques list and how they can transform the way you communicate with your customers. But first, let’s examine why NLP is so essential to the modern-day business landscape.

Why Is NLP So Important?
In a world of Google and various search engines, customers nowadays expect to type in a phrase or even an idea into a search box and instantly see personalized recommendations that are unquestionably relevant to what they intended to discover. It’s the type of interaction that must go on a scale and speed that can’t be sustained by human employees alone.
Instead, doing right by customers requires computers and AI-powered systems that will constantly learn and develop insights into what customers want and what they mean. This is where natural language processing can make the load considerably lighter for businesses in delivering exceptional customer experiences through better communication with clients.
As a result, your customer service representatives will be able to enjoy highly automated processes and stop performing repetitive tasks like summarization, customer support ticket classification, and even resolving uncomplicated issues.

However, when it comes to the biggest reasons why NLP advanced techniques are so crucial to modern-day businesses, it all comes down to improving bad site searches as they usually mean lost customers and the help that NLP technology can provide to your company regarding making sense of all that unstructured data that’s created during multiple points of communication your clients’ have with your business.
Below, we’ll discuss the five key NLP techniques that NLP specialists use to implement natural language processing into businesses’ day-to-day activities.
The 5 Major NLP Techniques That Can Truly Power Up Your Communication Efforts
Named Entity Recognition
Named entity recognition is perhaps the most popular and primitive algorithm in the sphere of NLP. This NLP technique extracts the core entities that are present in the text, which at the same time are the fundamental themes in the text. Entities could be names of companies, names of people, monetary values, dates, quantities, time expressions, locations, and other essential information found in the text.
The named entity recognition technique focuses on identifying and categorizing entities into pre-classified groups. For example, consider the following sentence:
- The temperature in Worcester, Massachusetts, is close to 90-degrees on this sunny June day.
From this piece of information, the named entity recognition NLP technique would categorize: Worcester, Massachusetts as location; 90-degrees as temperature; and June day as the date. This technique is solely based on basic grammar rules that companies use to manage large data sets efficiently.
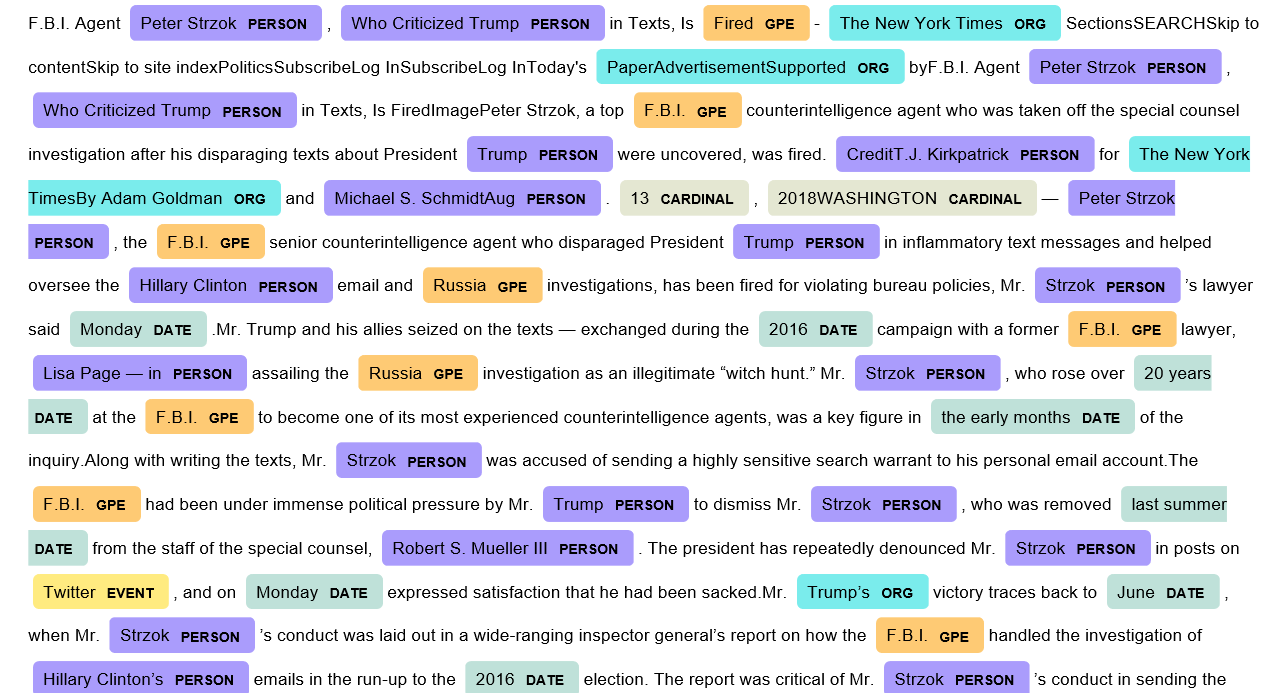
Stemming And Lemmatization
Stemming and lemmatization is a fantastic NLP technique to extract inferences of substantial data sets now that the size of data and information on the web is at an all-time high.
Stemming is the procedure of reducing inflected or derived words to their word stem, base, or root form. For instance, what stemming does is basically cutting off all the suffixes and prefixes of words, so after applying a step of stemming on the word “singing,” it becomes “sing,” or “produced” becomes just “produce.”
Lemmatization refers to doing things with the proper use of vocabulary and morphological analysis of words, typically aiming to eliminate inflectional endings and return the base or dictionary form of a word known as the lemma. Simply put, lemmatization deals with the lemma of a word that involves reducing the word form after understanding the part of speech or context of the word in any text.
Natural Language Generation
Natural language generation, or NLG, is another one from the long list of NLP advanced techniques that use raw structured data to convert it into plain English or any other language. NLG, which is also known as data storytelling, is very helpful for many companies that deal with large amounts of data as it can convert structured data into natural languages for a better understanding of patterns and gaining detailed insights.
The NLG technique can make data understandable to all by making reports that are solely data-driven like any kinds of reports, meeting memos, reports on product requirements, customer service inquiries, etc.
The natural language generation NLP technique has many stages, including content determination, document clustering, aggregation, lexical choice, referring expression generation, and realization.
Sentiment Analysis
Sentiment analysis is also one of the most prominent NLP techniques out there. With sentiment analysis, which is also known as emotion AI or opinion mining, you can understand the emotion or feel behind the written text.
The primary undertaking of sentiment analysis is to find whether expressed opinions in any text, sentence, document, social media post or review are positive, negative, or neutral, which is also called finding the polarity of the text.
Sentiment analysis is a perfect technique for empowering your customer service efforts because it usually works best on subjective text data rather than objective ones. In general, objective text data are either facts or statements which don't hold any emotions or feelings. On the contrary, the subjective text, like customer support inquiries, is usually written by humans showing feelings, affections, and emotions.

Sentence Segmentation
The primary task of this NLP technique is to divide all text into meaningful phrases or sentences. This task includes identifying sentence boundaries between words in text documents.
And because almost all human languages have punctuation marks that are presented at sentence boundaries, sentence segmentation is also referred to as sentence boundary disambiguation, sentence boundary detection, or sentence boundary recognition.
Why are Transformer Models Different To Older NLP Models?
NLP as a potent machine learning tool is utilized to augment human teams and help businesses find an edge in the ultra-competitive market. Moreover, NLP models like the latest Y Meadows software, which employs an entirely new infrastructure around NLP called “transformer models,” can help derive even more meaning from incoming written communications in a way that’s most valuable to your business.
In brief, a transformer is a deep learning model that adopts the mechanism of attention and evaluates the influence of different parts of the input data. This model is mainly used in NLP, and Y Meadows’ NLP solution embraces transformers to handle sequential input data, like natural language, for tasks such as text summarization or translation. Unlike older NLP models, transformers don’t require sequential data to be processed in a specific order. For instance, if the input data is a natural language sentence, the transformer doesn’t need to process the sentence's opening before the end. Alternatively, the transformers model identifies the context that confers meaning to a word in a sentence and allows for much more parallelization than older NLP models, saving time and money along the way.
Final Words
In this blog post, we’ve covered five of the most utilized natural language processing techniques.
However, there are other techniques worth mentioning also, like aspect modeling, topic modeling, natural language understanding, text summarization, decompounding, and more which are also very helpful ways to understand human languages more clearly for computers.
Once the information is extracted from unstructured data using the above-mentioned techniques, it can be directly consumed or used in machine learning models to enhance their precision and performance.
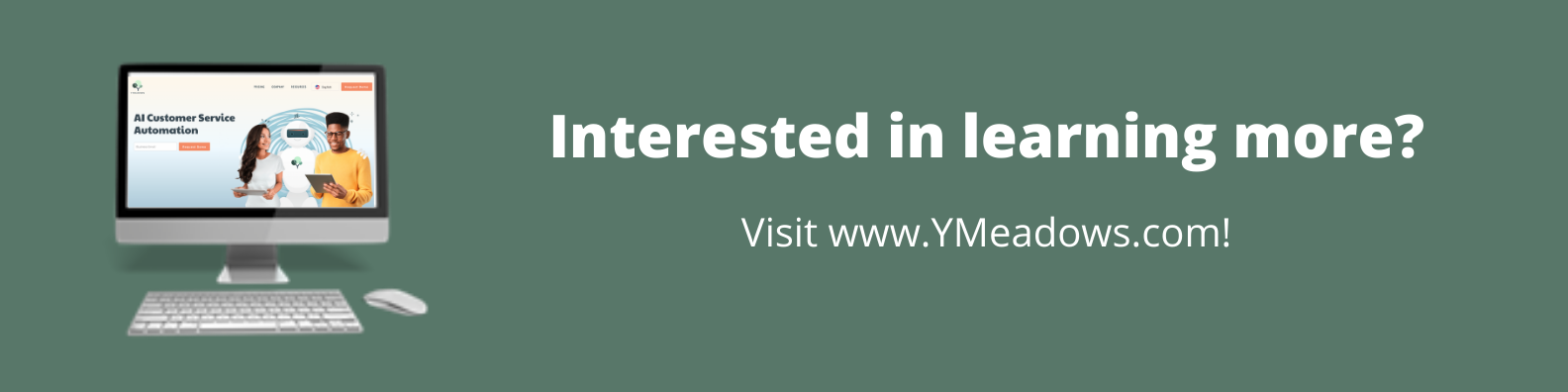
You Might Also Like
Explore how AI transforms chaotic support environments into organized, efficient systems, enabling companies to deliver better service.
Read more →Discover how AI is transforming customer service, making every customer feel like a VIP through personalized interactions, 24/7 support, & emotional insights.
Read more →It's one thing to be aware of the latest consumer trends, but the real value lies in effectively implementing them.
Read more →