May 21, 2021
The Evolution of AI Technology
Summer Terry

Although the concept of AI can be traced as far back as 380 BC in what is referred to as “mechanized human thought,” it was not until the 1950s that the field of AI as a research-based science was born. Over the next four decades innovations in AI technology were made through developments in programming languages, robotics, and automation. In addition to these technological advances, film depictions of machines with AI capabilities in the latter half of the 20th Century popularized the notion and increased interest among the general public.
By 2010, innovations in AI had made a significant impact on our daily lives. For example, smartphones with voice assistants and computers with “intelligent” functions had become the norm. Over the next two decades, rapid AI growth continued with breakthroughs in technologies leading not only to new commercial products but to applications directed toward improving businesses as well. During the 2010s these breakthroughs were primarily related to vision enabled technologies.
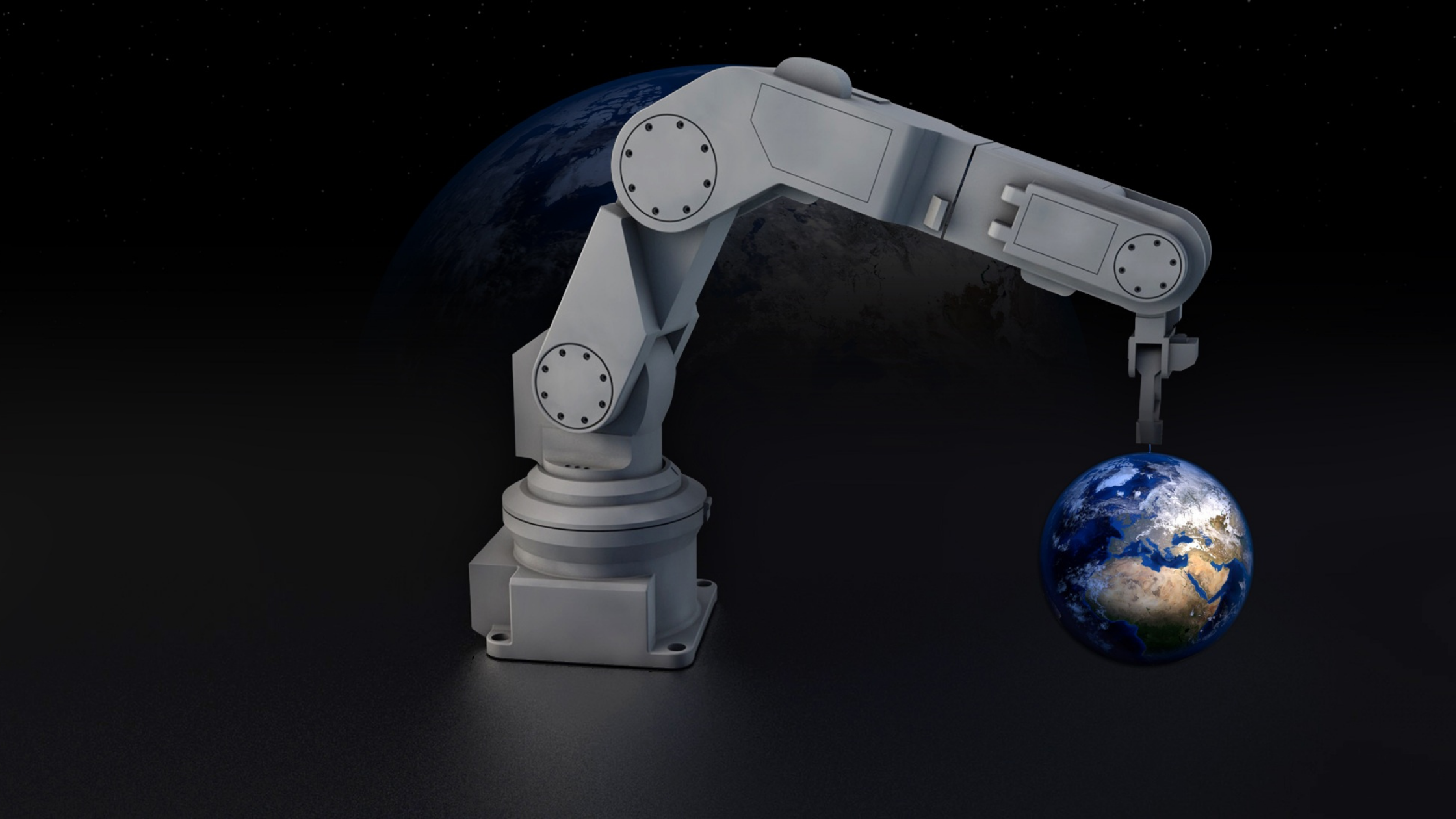
Growth in Vision Related Technologies
The first vision related breakthrough of 2010 was Microsoft’s launch of Kinect. Kinect had the ability to sense human body movement using only a 3D camera and infrared detection which quickly garnered praise for its human motion capture technology. Although Microsoft subsequently discontinued its Kinect gaming application, a non-gaming version, Azure Kinect is available today. Azure Kinect is a developer’s kit targeted toward enterprise users providing machine learning applications with connected sensors allowing users to create interactive virtual reality experiences.
Over the next few years, major advancements in vision were recognized through the ImageNet Large Scale Visual Recognition Challenges. This challenge was an annual competition held between 2010 and 2017 which used the ImageNet dataset to measure the accuracy of objective recognition and classification software programs. The goal of the challenge was to promote the development of improved computer vision techniques. It allowed for the measurement of progress in computer vision for large scale image indexing for retrieval and annotation and for the evaluation of algorithms for image classification and object detection at large scale.
The 2012 ImageNet Challenge was won by AlexNet, a convolutional neural network (CNN) designed by graduate student, Alex Krizhevsky. AlexNet dominated the competition achieving an error rate on ImageNet visual recognition of 15.3 percent which was half the previous error rate and more than 10.8 percentage points lower than the runner up. This success was due to the designer’s use of graphics processing units (GPUs) to process deep neural networks which increased processing speed and improved algorithm accuracy. For example, the neural network taught itself to identify cats with a 74.8 percent accuracy and faces with 81.7 percent accuracy from analyzing YouTube videos.
In 2015, further advances in CNN technology led to machines from Microsoft and Google beating humans in image recognition at the sixth edition of the ImageNet Large Scale Visual Recognition Challenge. Due to deep learning algorithms, the machines were able to outsmart humans in over 1,000 categories. The algorithms were based on different versions of artificial neural networks which performed in a similar fashion to the human brain. This breakthrough allowed machines to automate tasks that involved recognition of an object or person and then to make a decision about how to proceed based on that recognition.
Eventually, innovations in CNN applications led to the rise in popularity and use of deep learning neural network techniques. Deep learning techniques have subsequently been shown to outperform previous machine learning techniques across the computer vision field. Applications have included: improved web image searches such as Google’s Image Search; face recognition such as Google’s FaceNet and Facebook’s DeepFace; and object detection for purposes such as medical image analysis and diagnosis, and detection of defective parts in manufacturing.
Growth in Language Related AI
In addition to vision enabled AI advances, breakthroughs in language technologies emerged toward the end of the decade. These breakthroughs are expected to provide the groundwork for major advances in language based technologies during the next phase of AI growth. In fact, the Global Natural Language Processing (NLP) Market which was valued at 10.72 billion US dollars in 2020, is expected to grow to 48.46 billion US dollars by 2026.
In 2017, ‘Attention Is All You Need’ was published. This paper introduced the Transformer, a new simple network architecture model which allows machines to understand languages in ways that were not previously possible. Transformers utilize the mechanism of attention to weigh the influence of different parts of input data. The Transformer technology is significant because unlike recurrent neural networks (RNNs), Transformers do not require sequential data to be processed in order. This is because the attention operation identifies the context for any position in the sequence. The model has primarily been used in the field of natural language processing (NLP), but has recently gained popularity in other applications as well.
After the introduction of the Transformer model, major technology companies began to take advantage of its capabilities. For example, you may have heard of BERT, Google’s Transformer model. In fact, Google is not alone in recognizing the value of this technology. Microsoft, Facebook, OpenAI, and Alibaba have followed suit with their own Transformer models.
It is important to note that previous language processing models used hand coded rules and mathematical techniques. As time progressed, the use of artificial neural networks (ANN) to perform language processing became increasingly popular. This is because ANNs can learn from raw data which requires far less routine data and labeling.In the last few years, Transformer technology has continued to garner attention due to its many capabilities. For example, Transformer models can perform almost any NLP task. They can be used for language modeling, translation, or classification. In addition, they can help produce press releases, technical manuals, short stories, songs, and even computer code. Transformer models do these tasks quickly by removing the sequential nature of the problem. Only a few years ago, this was an impossible task for technology. But now, with new developments and applications, Transformer technology has the ability to transform businesses.
Final Thoughts
In order to benefit from current and future innovations, businesses must prepare. It is important for businesses to not only evaluate IT resources but human resources as well. In other words, companies must redesign their business processes to incorporate both humans and machines. This process is the key to maximizing the potential benefits of AI technologies.
The first step in this process is for companies to analyze the different tasks in current employee roles in order to identify those that can be automated through AI. In addition, companies should look for areas where AI can augment and enhance human productivity and creativity. According to Harvard Business Review, these are the areas of greatest opportunity for companies utilizing AI technologies. So, while professionals across industries will benefit from AI’s automation of routine tasks, they will also be able to rely on AI to help develop paths of thought for more critical communications. For example, communication professionals can use AI to generate ad copy or social media content while customer service agents can use AI to generate preferred practices in response to critical customer queries. In addition, company scientists can use AI to generate graphs and improve basic research and experimentation. Thus, the different ways that AI can augment businesses across disciplines and industries is limitless.
Keep in mind, this is not the time to wait and see what happens. This is the time for those who do not want to fall behind to gain an understanding of AI technologies and how they can enhance your business. Starting the preparation process now is key to realizing the benefits of AI.
In May 2021, Y Meadows announced the launch of its AI customer service automation solution. This solution empowers customer support agents by reading incoming customer communications and intelligently directing them to the appropriate resource, enriching the customer’s ticket with information that will assist the agent, or resolving the ticket by responding with the appropriate information automatically. Businesses that adopt this software solution early on will get a head start on redesigning jobs and improving customer experience. And, over the next few years, you can expect these businesses to find more opportunities for innovation, putting them even further ahead.
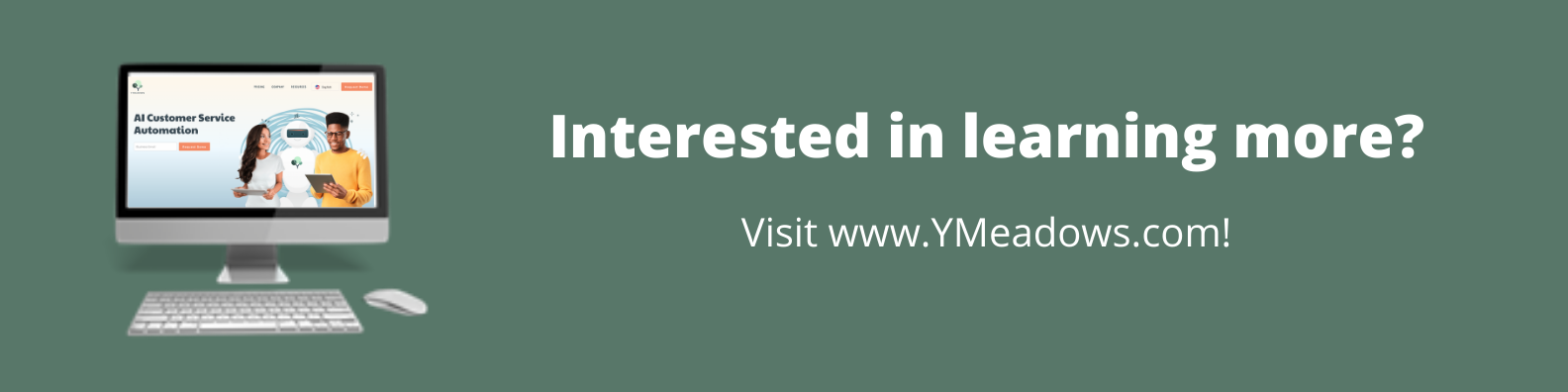
You Might Also Like
Explore how AI transforms chaotic support environments into organized, efficient systems, enabling companies to deliver better service.
Read more →Discover how AI is transforming customer service, making every customer feel like a VIP through personalized interactions, 24/7 support, & emotional insights.
Read more →It's one thing to be aware of the latest consumer trends, but the real value lies in effectively implementing them.
Read more →