June 2, 2021
Why A Chatbot Is Not Customer Support Automation
Summer Terry

What Are Rule Based Chatbots?
By now, most customers have likely had at least some interaction with chatbots. These text based tools became increasingly popular after Facebook launched its Messenger platform that allowed businesses to perform automated customer support. Chatbots such as these are considered to be rule based chatbots which are also known as decision tree chatbots.
As their name suggests, rule based chatbots answer questions using a set of rules on which they have been trained. These rules determine the types of problems the chatbot is able to handle. Depending on the customer issue, rules can either be simple or complex. But, it is important to note that chatbots can only solve simple problems.
This is where the major limitation of rule based chatbots lies. Chatbots are unable to solve complex requests and are susceptible to misunderstanding what the customer is trying to say. For example, “Where is my order?” or “Can I make a reservation?” are both simple questions that a chatbot is capable of answering. But, it is important to note that although a chatbot may understand the question “Where is my order?”, it might not understand the same question asked in a different way. In other words, if a customer does not explicitly match the language that the chatbot was trained on and instead asks “Is my package delayed?” the chatbot might not be able to accurately identify the intent of the question as ‘order status’. Thus, chatbots must be trained using variations of commands and phrases making it almost impossible for developers to write rules to cover every variation of each possible request.
Oftentimes, rule based chatbots have one of two main functions: a sales function for garnering contact details and scheduling calls or meetings and a support function for providing generic information like a website link. Similar to a decision tree or flowchart, rule based chatbots map out conversations. They either give the customer a set of predefined options that lead to the desired answer or predict the customer’s intent based on keywords and reply accordingly. However, the chatbot cannot answer questions outside of the defined rules and their performance depends greatly on how well they have been trained. In addition, sometimes chatbots are keyword based, which means they only react to specific words. These chatbots are limited by typos and are at risk of providing incorrect answers, causing negative customer experiences. Lastly, rule based chatbots do not learn through interactions and cannot improve without additional training.
Chatbots and User Experience
If you have never received an error message when interacting with a chatbot, consider yourself lucky. Unfortunately, users are often confronted with messages like “Sorry, I didn’t understand that.” or “Let’s try again. Can you say that in a different way?” Thus, user experience is often alinear with rule based chatbots.
If a user says anything that does not align with the rules the chatbot was trained on, it will get confused. For example, a chatbot designed to help people find general information like store hours and location will not know how to respond to a customer asking for the price of a product. Once a chatbot is confused, it will likely repeat the same set of questions until it is able to understand a response. Unfortunately, this scenario is quite common. In fact, according to a survey from Uberall, when asked what elements of chatbots need improvement, 43 percent of respondents said their accuracy in understanding the customer’s intent, 27 percent said their ability to get a human customer representative involved when needed, and 19 percent said their ability to hold a natural conversation. The same survey revealed that 69 percent of respondents believe the best potential use of chatbots is for tasks other than customer service support. Lastly, the survey found that 60 percent of users lack a strong enthusiasm for chatbots.
After interacting with chatbots, users commonly complain about 4 main factors. These factors include information overload, under or over developed personas, lack of added value, and malfunctioning problems.
Below, we will discuss each and how they have negatively impacted the value of chatbots as a customer support tool.
Information Overload
Research has shown that human ability to make decisions and remain productive is greatly diminished when dealing with too much information. Information overload refers to the difficulty in understanding an issue and making decisions effectively when you have too much information about that issue. Instead of initiating a productive conversation with users, some overzealous chatbots have the tendency to overwhelm customers with floods of text. When chatbots bombard customers with information, they become stressed, less engaged, and are likely to abandon the webpage. It is important to note the purpose of chatbots is to provide 24/7 service to customers but when customers cannot easily identify solutions they become useless.
Under Or Over Developed Personas
Creating the perfect chatbot persona has proven to be difficult for many software developers and customers have noticed. A major complaint with chatbots is their inability to mimic human speech and interact with customers. Although chatbots are essentially digital robots, they should not sound like one. For example, a chatbot who comes off as cold or stale will likely deter customers. On the other hand, overly humorous chatbots can have the same effect. Ultimately, a chatbot’s persona should match its brand image while also sounding empathetic and personable. Unfortunately, this has proven to be a difficult task to achieve.
Lack of Added Value
Although there are thousands of chatbots now available on the web, not all of them are useful. Some chatbots lack a purpose, strategy, and goal. When this happens, chatbots do not perform well or add value. For example, if the purpose of a chatbot is to provide customer support but only replies to inquiries by saying “Contact customer support during business hours” then it is useless. Unfortunately, this is occurring more often due to businesses implementing chatbots where they are not needed.
Malfunctioning Problems
The most common complaint among chatbot users is malfunctioning chatbots. When scripts break and programs do not perform the way they should, issues arise. For example, a chatbot that draws replies from if/then scripts will likely be confronted with a request that was not accounted for. When this occurs, chatbots attempt to recover by redirecting the conversation back into its predetermined parameters. The problem with this is that corrective questioning often leads to conversational dead ends.
Based on these factors, chatbots clearly have not lived up to expectations as a means of providing an around the clock positive customer experience.
So, if chatbots are not the best solution for automating customer support, then what is?
Y Meadows’ enterprise customer service automation solution
What Is Y Meadows’ Customer Service Automation Solution?
Y Meadows is a company dedicated to empowering customer support agents, increasing support team’s productivity, and improving customer experience. Their enterprise solution utilizes advanced AI, machine learning, and natural language processing (NLP) technology to handle customer inquiries and empower support agents. The software solution can organize and triage incoming customer support tickets, decrease response time by eliminating the need for agents to gather data from multiple systems, and analyze a customer’s request to instantly respond with relevant information and attachments.

Y Meadows’ Software Solution VS Chatbots
Y Meadows software solution varies greatly from chatbots. In comparison, Y Meadows’ solution uses machine learning to understand the context and identify the intent of a customer’s ticket before formulating a response. This is different from rule based chatbots because instead of using a set of rules to answer questions, the software focuses on understanding the context and intent of the incoming communication to develop an effective solution. In order to do this, the software was trained on a neural network which has the ability to think on its own after being trained on a vast amount of data. In addition, Y Meadows’ solution has the ability to generate answers to more complicated requests due to the use of natural language processing. The more customers use and train Y Meadows’ software, the better it operates with the user. This is because Y Meadows’ software learns through interactions and can improve on its own.
Y Meadows’ customer service automation solution is built for enterprises and can serve companies who have a large amount of data and incoming requests. Overall, Y Meadows’ solution is a much more effective choice for automating customer service. A few benefits include its ability to learn from information gathered, continuously improve as more data comes in, understand patterns of behavior, understand multiple languages, and have a broader range of decision making skills.
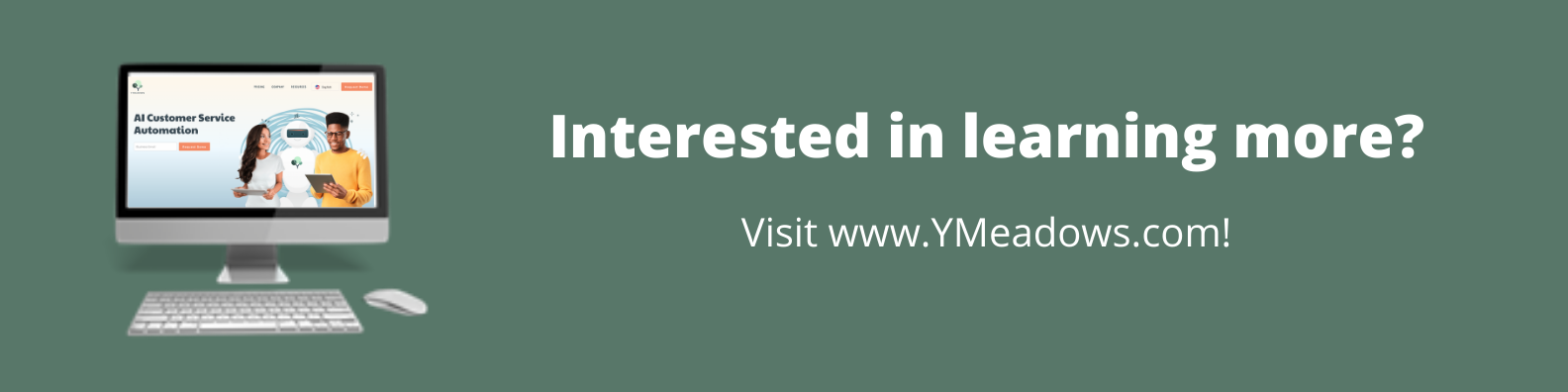
You Might Also Like
Explore how AI transforms chaotic support environments into organized, efficient systems, enabling companies to deliver better service.
Read more →Discover how AI is transforming customer service, making every customer feel like a VIP through personalized interactions, 24/7 support, & emotional insights.
Read more →It's one thing to be aware of the latest consumer trends, but the real value lies in effectively implementing them.
Read more →